The use of artificial intelligence (AI) in healthcare risks perpetuating historical bias. But with the right processes in place, AI can help solve healthcare bias problems rather than prolong them.
From the start of the COVID-19 pandemic, Black individuals and other people of color in the U.S. have been roughly twice as likely to die from the disease as have White people. And this is not only the case in America, but also in the UK, and it is believed to hold (though the data is harder to come by) elsewhere in Europe.[1]
Why? While a number of factors play a role, from lifestyle to housing to employment, one significant reason for the disparity is that Black people are less likely to be vaccinated.[2] And they are more vaccine-hesitant due to long-term distrust of the medical establishment. Here’s just one example of what feeds that mistrust:
“In 2019, an algorithm that helps manage healthcare for 200 million people in the US was found to systematically discriminate against Black people. According to research published in the journal Science, people who self-identified as Black were given lower risk scores by the computer than White counterparts, leading to fewer referrals for medical care.”[3]
Clearly, that’s the opposite outcome of equitable, high-quality healthcare. How can we use data and AI to combat bias in healthcare rather than perpetuate it?
The Data Dilemma
It’s easy to see why machine learning would be biased when you break it down to its simplest level. AI is based on algorithms, which are formulas using bits of information that can be combined with one another in order to assess a patient or a condition. If the pieces of information are incomplete or inaccurate, then the results will be flawed (commonly expressed as “garbage in, garbage out”).
The Color Code podcast shared the example of an algorithm for arthritis in people’s knees. “When a radiologist looks at an x-ray for arthritis, they see how small the joint space has become with age, or whether there were any new bony projections that could be signs of disease. Using that information, they would score just how bad that person’s arthritis is: 0 being the best, and 4 being the worst. That scale is called the Kellgren-Lawrence system.”
However, that scale is based on data from only one group of people who all lived in the same area and were the same gender and race. How can that data truly represent a global population of all ages, races, and genders? It can’t.
On a larger level, lack of information affects all information. If a certain population, for example lower-income families, tends to seek medical care less often, that data is simply missing. Any AI built from that data will be flawed.
We know artificial intelligence has huge advantages in speed, scope, and scale. It can truly be called a game-changer. But given the data challenge on both sides — what we provide as individuals and how the system responds — how can healthcare overcome bias?
Three Steps to Lessen Bias in Healthcare AI
It’s not as simple as saying, “fix the programming” or “fix the data.” How do you fix it? What parts of it? Who makes the decisions? Given the real-life ramifications of making the wrong decisions, reducing bias must be thoughtful, deliberate, and ongoing.
First: Link Data Goals to Organizational Goals
The first step is to link your project to organizational goals, and then look for AI solutions to help support them, rather than making AI a stand-alone project.
“The KPIs you’re defining for your departments could very well include specific goals around increasing access for the underserved. ‘Grow Volume by x%,’ for example, could include, ‘Increase volume from underrepresented groups by y%.' That’s a solution that AI has to help solve; it would be difficult without it.”
- Chris Hemphill, VP of Applied AI and Growth, Actium Health
To determine the right metrics for your healthcare system, look at your patient population and your community. What’s the breakdown by race and gender of your patients versus your surrounding communities? That’s one practical way to put a number and a size to a healthcare gap.
Second: Diversify the Decision-Makers
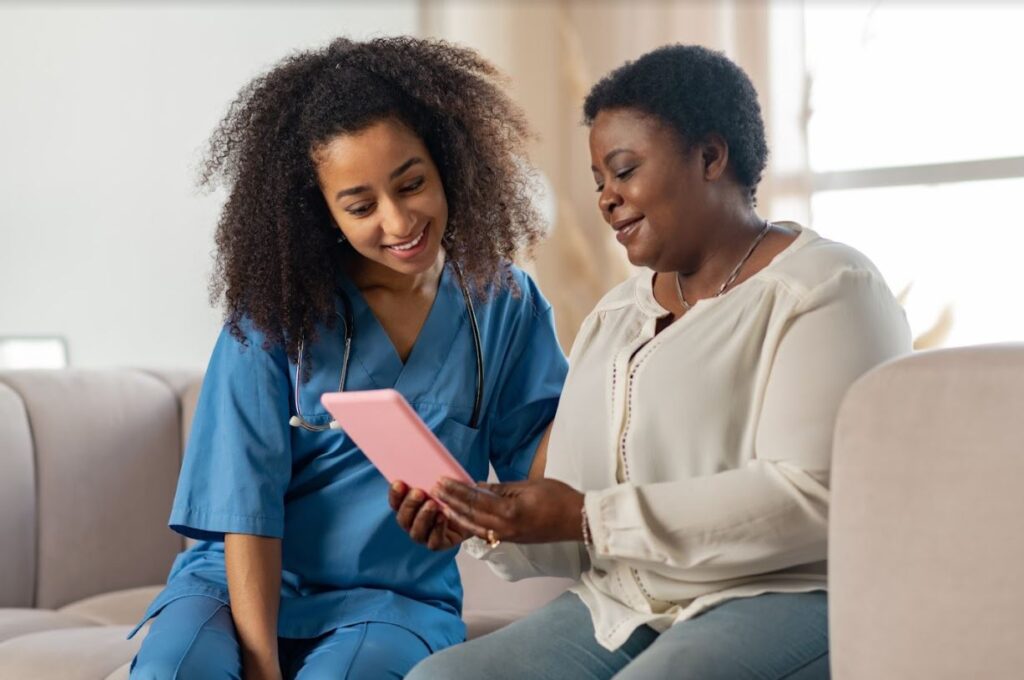
It’s often said that if the people at the table don’t include the people you’re designing for, then the solution will fall short. Create a diverse team, and look beyond the visible — gender, ethnicity, age, and physical ability — to include socio-economics, neurodiversity, and different personality types.
The more you can bring different perspectives to the project, the more you’ll move beyond the “bubble” we each live in, and the more you’ll be able to identify assumptions. Numerous studies and books have been written to examine this phenomenon of diverse thinkers producing better results. For example, look at the classic Netflix algorithm challenge when “unrelated teams from different professions around the globe joined forces [and] beat the company’s existing program for predicting users’ movie ratings based on previous ones.”
It can take longer to arrive at decisions with a variety of perspectives, but upfront challenges to data are far better than gaps after a process has been built. For example, now we know that heart attacks present differently in men than women, we can ask different questions. But for decades we didn’t, so we focused on symptoms like stabbing pain instead of also asking patients about fatigue, shortness of breath, or nausea.
Third: Always Put Yourself in the Patient’s Chair
Think of the last time you were filling out a survey. Did you fill in all the demographic data? Or did you skip it, thinking, “They don’t need to know my income,” for example. People generally distrust “authority” and certain demographics in particular distrust healthcare. To alleviate this distrust, be clear about why you’re asking for particular information and exactly how you’ll use it.
A simple sentence can help. For example: ”We’re asking your age because certain health care risks are higher at different ages,” or “We ask your assigned gender because medication acts differently in men versus women.”
Healthy relationships are based on trust, which has to come from mutual understanding. The more we can remember that when gathering data and asking questions, the more likely we’ll be to provide more context and clarity.
The Benefits of Eliminating Bias
Healthcare as a profession is built on data, both observed (patient appears disoriented) to self-reported (how intense is the pain?). That’s true both for diagnosis and for treatment, as well as for outreach. For example, if your service line marketing is trying to attract more cardiology patients, but the algorithms are based only on data from men versus women, you’ll miss many patients who would benefit from early intervention.
We all want to help: that’s why many people choose healthcare as a profession. None of us want to miss a symptom or make the wrong diagnosis based on missing data. And we know that if we aren’t reaching all those who could benefit from a particular type of treatment or prevention, our business isn’t fulfilling its purpose.
As Dr. Zachary Hermes of Brigham & Women’s Hospital puts it in a Hello Healthcare podcast, health equity is both a “tug at the heart” issue as well as a business issue. It’s not simple, and it’s not a once and done fix. But with continuous focus and prioritization, we can deliver better outcomes for more people – the ultimate “why” for reducing bias.
For more, listen to the Hello Healthcare episode, “Where to Start with Health Equity.”
Sources:
[1] A Brief History of Racism in Healthcare
[2] Why Black Americans Are Less Likely to Take the COVID Vaccine
[3] A Brief History of Racism in Healthcare