Join Dean Browell (Chief Behavioral Officer, Feedback), Danny Fell (Sr. Strategist, Optum), and podcast host Alan Tam as they discuss the concepts of quantitative and qualitative data for healthcare marketing. They dive into the importance of combining both data types for a more comprehensive understanding of the patient and driving them to care. The trio covers both pitfalls and strategies for success, highlighting key use cases and the need for human involvement in interpreting data. The conversation also touches on the role of technology, AI, and the current state of data-driven healthcare marketing.
You don’t want to miss this episode!
This conversation is brought to you by Actium Health in partnership with the Forum for Healthcare Strategists.
Subscribe to receive emails when new episodes are released.
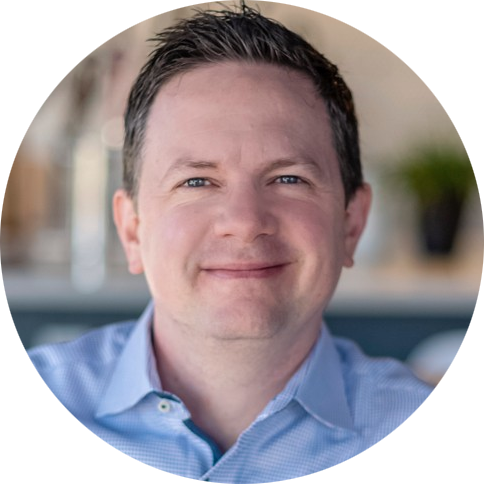
Dean Browell
Chief Behavioral Officer
Feedback
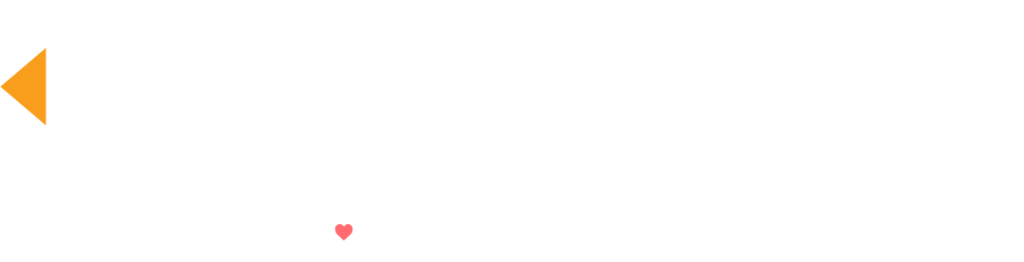
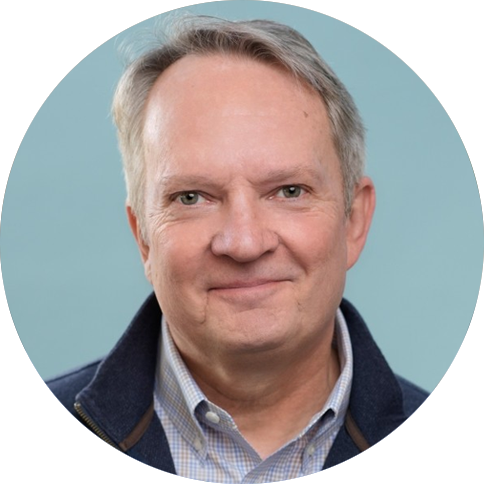
Danny Fell
Senior Strategist
Optum
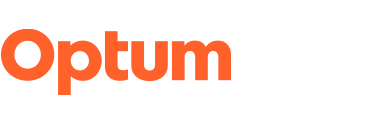
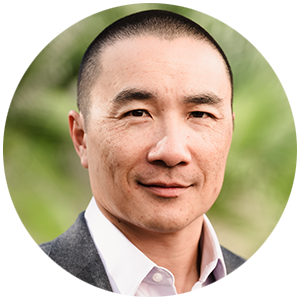
Alan Tam
Chief Marketing Officer
Actium Health
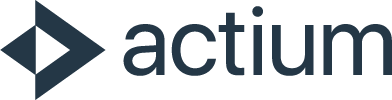
Danny Fell (00:00):
The importance of having both qualitative and quantitative data in what you’re doing in marketing because you get so much more value and you risk losing perspective, especially today, with so much hype around big data, AI, things that are more quantitative, but you lose the richness of the qualitative insights.
Alan Tam (00:33):
Hello, Healthcare. For those of you that have been listening to the podcast for a while, you probably know that data driven marketing is something that I’m personally extremely passionate about. It’s been a great topic of exploration and discussion with many of our esteemed guests. Today, I’d like to dive a little bit deeper in that realm by examining the powers of quantitative and qualitative data. Some say that when you combine the two, the possibilities are endless. I have the utmost pleasure today of having two amazing thought leaders in the space joining me, Danny Fell, senior strategist at Optum, and Dean Browell, chief behavioral officer at Feedback. What a treat it is to have the both of you on this podcast today.
Danny Fell (01:16):
Hey, thanks for having us on.
Dean Browell (01:17):
Absolutely.
Alan Tam (01:18):
So for those of you that aren’t familiar with either of you, why don’t we just start with a little quick intro in terms of what you both do?
Danny Fell (01:25):
Yeah. Why don’t you start?
Dean Browell (01:27):
Sure. So I’m the chief behavioral officer at Feedback, which I realize is this very lofty title that doesn’t necessarily tell you what I do. And we’re a digital ethnography firm, so ostensibly, social listening through behavioral science. And so I get to both present the data often and sort of the thought leader within the company and one of the founders. We’ve been around about 13 years.
Alan Tam (01:50):
Amazing.
Danny Fell (01:51):
And I work for Optum in our analytics group and work with hospital systems and healthcare providers to tap into some of the solutions and the data we have, and been with Optum for about four years.
Alan Tam (02:05):
That’s great. So who’s quantitative and who’s qualitative here?
Dean Browell (02:10):
We keep joking it’s like I’m a Mac, I’m a PC ad.
Danny Fell (02:12):
Yeah.
Dean Browell (02:14):
I’m qual.
Danny Fell (02:16):
And I’m quant.
Alan Tam (02:18):
So for our audience, what is quantitative data and what is qualitative data? Let’s start there.
Danny Fell (02:23):
All right. So I’ll take the quant one, and then you can explain yours. Pretty much anything that is a relatively large set of data becomes quant. Right? At some point, you want to be able to have a measurable set of data. It doesn’t have to be big data, which was the title of our talk yesterday, and there is no real threshold between what is officially big data. Typically, when you start talking about big data, it’s quantitative data this is large enough that it requires some tools or computers to be able to analyze the data or work with the data. But the difference I think between qual and quant, I’ll be interested to hear what you say, is we’re more about using numbers and being able to draw some type of statistical or measurable difference in the data, which is a little bit different from qual. Yeah.
Dean Browell (03:18):
Yeah. At its heart, qualitative is really about the observation of behavior. So for example, interviews, interviews are.
Danny Fell (03:29):
Could be qualitative, we’d be qualitative.
Dean Browell (03:29):
This is qualitative, yeah. Focus groups, things like that. Now what we do day to day is use a qualitative lens to look at social listening. And I should point out, just to say it, qualitative can be come quantitative when you have enough of it, enough interviews, enough focus groups, or in our case, enough behaviors that we’re observing. You can code those and they can get into the numbers realm in terms of getting quantitative. But really, it’s about … And this is what I fell in love with in my early PhD work, was ethnography, this idea of observing and then writing these rich descriptions of what you’re seeing to really get a good sense of the why behind things. Quantitative is incredible at telling you what’s happening. It can’t … There are definitely limitations I think about getting to the why. And so I think that qualitative for me often provides context.
Danny Fell (04:21):
Yeah. I think that’s a good way to describe it.
Alan Tam (04:23):
That makes a lot of sense. So earlier in my introduction, I talked about combining these two data sets. Should they be combined? And what are some of the advantages, as well as dangers potentially, of combining these two data sets?
Danny Fell (04:39):
It depends on what you mean by combine, obviously. If you’re trying to get some type of statistical measure, you would want to be careful in combining qualitative and quantitative data that you’re not maybe deriving incorrect assumptions or numbers that are based on really small counts that could be not project-able to the larger universe, for instance. But is there a lot of blend between the two? Sure. You gave an example, if you have enough qualitative data, it can become quantitative. Likewise, you may be doing quantitative things, phone surveys, online surveys, that as a part of that collects qualitative feedback. Right? So I’ve answered your 10 multiple choice questions, but I also wrote in some comments about what I like or don’t like about your product, so that would be qualitative sort of buried within the quantitative research, for instance.
Dean Browell (05:34):
And I think some of it too is sort of your approach, I mean, it’s one way to say mixed methodologies are always good because you-
Danny Fell (05:42):
That’s a good term.
Dean Browell (05:43):
Get at different things. But I also think that it’s not just for the sake of mixing it. It’s also this idea of, for example, I love often saying, “Zero is a data point.” Right? So if you don’t see a response that you were expecting, or maybe you didn’t get a particular demographic responding to a survey, why? What is that zero? And sometimes by combining them, you can get some better answers. Right? My favorite thing to talk about is NPS scores, net promoter scores. So would you recommend this brand? I would then argue, I don’t want to know just would you, I want to know do you.
(06:20):
And so for me, it would be not just how you answer on the survey, but then looking out and saying, “Does that demographic actually talk about you out loud, in the wild to peers and things like that?” And so I think there’s some interesting ways to be able to validate and give a more complete picture of what you’re looking for by if it’s not combining, at least utilizing both methodologies.
Danny Fell (06:44):
I think the premise of our talk yesterday was the importance of having both qualitative and quantitative data in what you’re doing in marketing because you get so much more value and you risk losing perspective, especially today with so much hype around big data, AI, things that are more quantitative. But you lose the richness of the qualitative insights.
Alan Tam (07:11):
That makes a lot of sense. What are some specific examples that you guys have seen in healthcare that actually utilizes both data sets in a meaningful way.
Danny Fell (07:22):
Sure. Do you want to start with qual?
Dean Browell (07:24):
Yeah. Well, I think, I mean this is pretty recent, just even the last week we did an interesting study where the preference data, the survey, preference survey for a particular hospital came back a particular way, seemed pretty glowing. But what we were finding on the social listening side was that people were actually a little more sour about a couple of things that we were looking at. And what was kind of fascinating about it was seeing how when you look within the market share, market share was low. So in some ways, the preference data wasn’t telling the whole story. People may be willing to promote and talk about this particular brand or system, but traditionally, they weren’t doing that.
(08:04):
And so it was just a way that working together, that qual and quant, really did give them the whole story that explained why the market share was so low because market share’s low, and you do a preference study and it’s very rosy, you can sort of be led to believe maybe it’s a better situation than it really is. And I think that thinking about it from a marketers point of view, what kind of messaging do we need to get out there and really understanding that audience, I think it’s that sort of good example I think where both sides really did complete a picture, but on their own, I think that it would always make you feel uneasy that you were missing a piece if you had just done one of those things.
Danny Fell (08:42):
Yeah. Here’s an example for the physician marketing folks in the audience. We have a tremendous amount of historical data on where people go for care, or types of care they’re getting, what physicians refer to what facilities, things like that. It tells us a lot about market share, referral patterns, but we don’t always know, to Dean’s point, the why behind that. So if you’re a physician marketing professional, or you’re someone who goes out and meets with and visits with those physicians, the feedback you get, the one to one, can be as important as, okay, I’ve got all this great claims data that tells me where business is going, but I don’t necessarily know some of the insights behind it.
(09:26):
On the quant big data side in healthcare marketing, we’re using it a lot. Right? We’re using it for predictive analytics. We’re using it for personalization. We’re using it for optimization of advertising and website traffic and things that we do with apps. And we use it to market for acquisition of new patients. So big data or large sets of data, however you want to define that, are being used in a lot of different ways in healthcare and healthcare marketing specifically. Yeah.
Alan Tam (10:04):
I think those are two amazing examples that you have both shared. But I think another integral piece to data, quant and qualitative, is kind of the role of technology. So my next question to both of you is: What is the role of technology in data driven marketing?
Danny Fell (10:24):
So technology is doing a couple of things. On the management side of data, the ability to use computing power, algorithms, business intelligent software, to analyze large sets of data has really come a long ways. Right? So when we’re talking about millions or hundreds of millions of data points, you need that kind of computing power to do that kind of analytics and derive information from that.
(10:55):
On simply the signal side of it, we have more people online using more devices, leaving more trails, bread crumbs. We have more technology that tracks what people do online. We have people using home monitoring devices, remote monitoring devices, so technology is throwing off a lot more data, which sort of perpetuates the need to manage more data. And that’s a challenge at the same time. So there are benefits of it, but also, it creates new and different challenges.
Alan Tam (11:32):
That makes sense. What are some of the common pitfalls that you guys have seen when applying technology and data and having that all work together.
Dean Browell (11:40):
I’ll take that one. Well, so my argument would be, and this is not just from the qualitative side, but just in general, is that having a human being involved. And part of it is to just gut check things, so that when you’re interpreting the context of things, you understand sarcasm. Right? You understand the context of the dates that are involved. What’s the timeliness of the data? And you just kind of have somebody that can keep the main thing, the main thing, for the mission of what you’re trying to gather.
(12:12):
From an example standpoint though, I think that’s where we talk a lot about personas in our presentation. And personas are a great example because often when you’re creating a new website, let’s say for a hospital system, quite often you would build personas out of the activity around the old website. So in other words, you’d look at-
Alan Tam (12:33):
Is that bad?
Dean Browell (12:34):
You’d look at the analytics of the old website and use that to build … How should be build the new website. The problem is, well, if you look at that from a different … Turn that prism a little bit, you realize that you are looking at data of people who you’ve already captured, so you’re not in any way looking at the behavior of those who you want, who you’re trying to draw. Two, it’s from a website that is bad enough, you want to replace it. So you know already that it’s not the optimal framework.
(13:03):
And so I think just having a human hand in there, understanding the limitations of the data, so that you can go get more. And I think that’s what we’re really getting at with our entire sort of concept around thick data, which is the idea of understand the limitations so that you don’t fall into those pitfalls, and you can fill those gaps with something that’s going to help you finish the last mile of what you’re trying to understand. And personas, I … I mean, there’s other pitfalls with personas just using generation, ways that you could segment very bluntly that isn’t helpful. But I think that it does speak to that idea of tech is great, but you can also lean on it way too hard.
(13:44):
And in our industry, our sort of subset of the industry of social listening, most of the open APIs that draw out just constant data are only to the bigger channels. That’s not bad. We want to know what someone who’s been diagnosed with breast cancer says on Facebook, understanding their journey, things like that. But for us, the real incredible behavior that’s worth knowing, that’s going to really make us understand their journey, is on breastcancer.org, a forum or message board that doesn’t have an open API. So you don’t want to get sort of shiny object blinded by the tech just because there’s this open API to not understand, that might not be where all the best information really is. So it’s just kind of going in eyes wide open with that.
Danny Fell (14:32):
I think there’s a big risk of that with quantitative data in particular. Right? So we get fixated on numbers. This is human behavior. Right? We can anchor people’s belief in putting out a number in front of them. We can distort how people think about things simply by throwing out statistics or percentages. Right? So I can say, “70% of an audience did this or said this,” and it sounds meaningful, and it almost sounds like the majority, which you would say is the majority, are doing that. But without also saying, “Well, there’s another 1/3 of people who aren’t doing that,” you sort of are missing part of the argument.
(15:15):
And so I think oftentimes, we, all of us can get sucked into … I think you mentioned fixating on a number or on what appears to be a statistical thing, as opposed to stepping back and saying, “Okay, let’s look at the big picture here. What else does this tell us about our audience, or our actions, or what we’re trying to do?”
Alan Tam (15:37):
I really like what you both shared just now as it relates to having a better understanding and looking at it from a more holistic perspective, and understanding the limitations of data, statistics, and the need for human involvement. And I think that’s something that’s really critical, which kind of leads me to the next question I have for the both of you, AI.
Danny Fell (16:01):
We don’t really need humans now, do we?
Dean Browell (16:03):
That’s right. We’re actually just simulations answering the questions here.
Alan Tam (16:08):
So AI is the talk of the town, ChatGPT. There’s fear, there’s adoption, there’s mixed feelings about it. How does or can AI fit in, in data driven marketing? Or does it? And what are some of the limitations there that you guys see? And I surmise that your answers are probably going to be similar to what you just talked about.
Danny Fell (16:34):
Probably. AI’s being used a lot. I think it’s been being used. We’ve been using it for years now. And a lot of people don’t realize the extent of AI in their smartphone, in their car, in their Alexa home voice activated devices. So AI itself has been around quite a bit, and AI is also a very general term. Right? It encompasses a lot of things. Our team works with machine learning to build predictive models. That’s a form of AI, but probably more appropriately called machine learning. Some of the newer technology, you mentioned ChatGPT, which is a generative AI technology.
(17:20):
I think the significance there is it requires large sets of data. Right? I gave the example yesterday that the GPT three version, there’s now four, but the three version was trained on something like 45 terabytes of data, which to put that in context is about three million books. Right? I think I looked it up. It’s about 10 times the size of the Austin Public Library. Right? So that requires a tremendous amount of data, but it is not necessarily going to be always accurate. It’s going to be influenced by what data you actually gave it, what rules you built around it, so there are some real limitations when we start to think about AI and specifically generative AI, and rightly so. There should be more structure around how we approach using some of that and more thought put into I think the ethical use of it, in particular, in some cases.
Dean Browell (18:25):
It really reflects, I think, the pitfalls we were just talking about, which is just having that hand on it to understand what the limitations are, so that it can be the most helpful. We’ve employed that kind of machine learning AI. We’ve experimented where in our process of social listening with the kind of behavioral lens, it might fit best. We’ve tried it on the front end. Problem with the front end is it’s not always great at predicting the kind of behaviors, such as what communities are formed around different diagnoses, for example.
(18:55):
So we also found that if we ask it to create personas, they’re very kind of clunky, incredibly general. I mean, actually, they’re not … It’s not that they’re not useful, but rather, they’re just way too broad. So what we found is that actually where it fit really well is after we’ve already created some broad personas of our own, from on hand with humans, and then let AI look at the data that we’ve segmented by persona. We were using Watson at the time from a machine learning standpoint. There was actually some really great insights from an emotional standpoint, being able to code for different emotions within those segments.
(19:37):
But it was interesting that it kind of took a human touch to create those initial segments. It wasn’t as effective at creating the segments as it was understanding once we got some commonalities in there, really digging in and pulling in some great insight. So I think the best way to think about it, at least from my perspective, is it’s another tool. I think, unfortunately, I think from a media perspective, we tend to talk about it as if it’s a new end all, be all, not just a tool. And I think that runs us into the dangerous category of: What do we allow it to do that we might rely too much on? But it also doesn’t put it in the right context.
Danny Fell (20:16):
Yeah. I think the context is really important, and there is a tremendous amount of, rightly so perhaps, attention on it right now, but also, a tremendous amount of hype. I think one of the interesting things is multi-modal generative AI, which is essentially not just text, which a lot of people have played with, or not just photos, show me a bear on a beach drinking a diet soda, but combining those, so where you’re getting text, images, perhaps video. That’s very exciting and also a little bit daunting in terms of what the computers are actually able to do, or the algorithms are able to do.
Dean Browell (21:00):
Wait until you see my photo of you with a bear on a beach drinking a diet soda.
Danny Fell (21:03):
Excellent, excellent.
Alan Tam (21:05):
So AI has been used I think for quite some time on a clinical side of healthcare, which I would say is much more risk averse than the non-clinical side of healthcare. And Dean, based on what you work on, I think there are remnants and sparks of AI that are starting to bleed into the non-clinical side of healthcare. What are your opinions on, is healthcare, the non-clinical side, ready for more AI?
Dean Browell (21:38):
Readiness is a fun term to think about that.
Danny Fell (21:43):
So I think one of the interesting challenges for the non-clinical side of healthcare is we’re under tremendous cost pressures. And so while the cost of a lot of this technology and data is coming down rapidly, it is still a big investment. Maybe it’s investment in data. Maybe it’s investment in technology. More often than not, it’s investment in people who understand how to use the tools or do the analysis. And so I think that there has to be a balance there in terms of understanding what type of investment should you be making, can you be making, and that’s going to limit what organizations can do. But yeah, I think the business side of healthcare, if you want to put it that way, is ready and will benefit from a lot of artificial intelligence applications.
(22:42):
Likewise, I think there’ll be a lot of things we throw stuff at the wall that don’t work. And that’s okay too. I think we’re very early in this, and so experimenting, testing, failing is okay, more so on the business side of healthcare, obviously, but I think we’ll see a lot of that as well.
Dean Browell (23:00):
I think the other thing to keep in mind is we’ve been here before, even just talking about big data generally, where there’s a lot of capital investment, and you may even have a lot of incredible data to make decisions with. But it’s all about whether you bother to try and make decisions with it. And so we do run the risk of AI being another Ferrari we have in the garage.
Danny Fell (23:21):
There’s a long history of that.
Dean Browell (23:22):
Either the people that you don’t have to use it, that maybe they end up being last and first out from the standpoint of cuts. So I actually try to be somewhat optimistic about a pessimistic situation. I actually think that it’s good that we’re having this conversation about AI during lean times because I think it’s probably going to make for smarter investments than if we had it during sort of a heyday, where people might invest an awful lot and then never actually operationalize it the way that it could be used.
Danny Fell (23:52):
Yeah. So you reminded me of a funny anecdote. I’ve been in healthcare a pretty long time. I can remember in my early days, we’d be touring a hospital marketing department, and this was early computer days. Right? And they would inevitably point to a computer in the corner that was sitting there unused and say, “Oh, yeah. We bought some planning data tool, but nobody actually uses that.” And you would see that in a lot of cases, and I think that’s exactly your point.
Dean Browell (24:23):
Or silos, I mean, that’s the other problem, is that one department buys it, or buys into it, invests in them, and then it just ends up behind kind of a locked door because the collaboration elements aren’t allowing it to be as beneficial to the whole organization. And that’s happening right now with big and small datasets already in healthcare. So I think hopefully this can be a good example, I hope, of how things could cross, knock down some barriers, but also just be used in a much smarter way, and we’re actually driving that car around, not just buying it and letting it sit there, especially with the high turnover we’ve got. I think that’s another problem.
Danny Fell (24:57):
The car can drive itself.
Dean Browell (24:59):
That’s true. Maybe it doesn’t need us.
Danny Fell (25:00):
Maybe you don’t need us.
Dean Browell (25:00):
That’s right.
Alan Tam (25:02):
So what would you say is the current temperature or state of data driven marketing in healthcare? Where are we on the spectrum?
Danny Fell (25:11):
Wow.
Dean Browell (25:13):
On the spectrum, I think.
Danny Fell (25:14):
We’re definitely on the spectrum. If you look at some of the industry research out there, American Marketing Association, Salesforce, others who are, Gartner, who are interviewing, talking to, marketing professionals, it’s all about data. The number of data sources are escalating. The pressure to use more data, the pressure to have more analytics expertise, I think it’s again, it’s a good thing. Right? I’m a data guy and believe in data driven marketing. But I think it also is potentially displacing other important things we’re doing, and so we have to continually come back to, okay, this is good. But what are we using it for? And what are the use cases that we want to drive from it? So I think those are all keeping it in context, as you said, I think is super important.
Dean Browell (26:11):
Yeah. I would just add that somewhat to the previous comments about silos, is that I think actually if we look at the data flatly, I think we’re in a good place. The problem is I think from an operationalized standpoint, we are not often in the most optimal place. In other words, we may have lots of data we could be using to make decisions with, but because of the way departments don’t talk to each other, or the data isn’t talking to each other, or in some cases, two people in the same office aren’t talking to each other. I think that we do run that risk of not being able to use it to its full potential. I think we’re in a kind of data rich scenario. I think sometimes we don’t know enough about it to know what our gaps are, and I think that’s where, again, I think it’s just a matter of evaluating what you have, what you could have, what you could connect, and then showing how all boats rise with it.
(27:04):
I think that’s where I think data, I feel, optimistically again, it could be kind of a unifier for buy-in across departments in a way that other industries may not always have that same feeling. So I think hopefully it can break down some barriers. We are in an unusual time because I do think when we first talked about this idea of talking about big data, thick data, that whole concept, there was this idea that everybody had bought into big data, but not necessarily were using it. And I don’t know that that’s changed a whole lot. I feel like some are using it more than others, but the silos are real.
Danny Fell (27:41):
Yeah. And consumers are facing this everywhere. Right? Now we don’t just have a thermostat that turns the temperature up or down, we have a thermostat that tells us 27 different things about our house. And so there is a tendency of society as a whole to lean into all of this data and these data points as well. But again, some of those are important, some are less important.
Alan Tam (28:10):
You guys have shared some amazing examples, really good examples of using that data. What are some bad examples that you guys have seen for data driven marketing, or they’ve failed?
Danny Fell (28:24):
So I’ll give an example, not necessarily a failure, but I think a good illustration of getting ahead of where maybe you want to be. I worked with a large health system that wanted to bring a tremendous amount of data into a platform, consumer data, millions of data points, but didn’t know what the use cases were, hadn’t defined the use cases for it to begin with. And so rather than pulling all the data in and then trying to figure out what to do with it, I think they smartly took a step back and said, “Let’s develop some use cases, figure out what data we want to start with. We can always add to it.” And that was a much better approach than simply dumping a tremendous amount of data into programs and then trying to figure out what to do with it because that’s not only time-consuming and costly, but again, it also has the potential to take your eye off what really is a key objective or a key outcome that you’re trying to achieve.
(29:30):
So I think that’s one example of where we can easily get sidetracked with kind of what’s in front of us. I call it the data buffet. Right? I’ll take one of those, and one of those, and one of those because I think it’s good. But you get back to the table and you didn’t really need all those, so that’s one challenge.
Dean Browell (29:51):
Yeah. I think cadence can lull you into a false sense of security too. We’ve had a conversation with an old friend who was at a very large health system. And they talk about doing the exact same study over and over again. They’ve been doing it for years. And it’s shown that there’s a problem, but they just have really no idea what the problem is. And this is put together around employee satisfaction, but it was this idea of maybe next year, we’ll get more insight into what the problem is, and the idea of just continually doing the same cadence until finally it was, “Okay, we should probably add some other element here to try and … We’ve got a lot of what and not a lot of why.”
(30:32):
And so I think part of it is that failure to get too comfortable with that cadence and not ask enough questions, I think that goes back to that human element. Right? Ask questions about your data. What’s not there? Zero is a data point, things like that, and not getting lulled into a false sense of security just because you’re doing the same routines or pulling the same data over and over again, but really asking some questions about what you might not be seeing.
Danny Fell (31:00):
Yeah. And there are some other practical things. Data governance is a really important topic for organizations to be addressing right now. And we have seen in the news nationally some problems with data and tracking technologies and things like that. And so organizations getting a better handle on: Where is our data residing? Who has access to our data? What are we doing with data points? I think that’s all really important, and it’s easy to get focused on the technology and not have some of those data governance elements in place. And so I think there’s a real need for that.
Alan Tam (31:42):
For folks that want to kind of get started with data driven marketing, hopefully they’re already doing data driven marketing, but combining quant and qualitative data, they’re not sure how to do it, what would be your suggestion in terms of where to start?
Danny Fell (32:02):
I would just ask ChatGPT.
Dean Browell (32:04):
Just ask them what they would say. I have two answers to that. Number one is I think just an audit of what you already have. What data’s already accessible? And maybe data that’s supposedly in an organization may be inaccessible because of a silo, but just try and do an audit of what it is you’ve got. And then again, look for those gaps. What could you use to fill those gaps to illustrate a little bit more? The other, let me give you a more practical example though, and we gave this answer to a question on the talk, I think you could for example, look at the data you’ve got. Maybe that’s patient data from a behavioral standpoint, where you’ve already got in-house, then do something qualitative and end with something quantitative.
(32:49):
The idea being that each informs the other. Look at the larger dataset that you have. Where are the gaps? Great. Do the qualitative to try and expose and give some clarity into what might be in those gaps. That also then helps you answer, ask better questions in the quant surveys that you might do. So it isn’t even that, it’s just inserting into your process some element that kind of helps you check yourself with a mixed methodology, but makes you ask the right questions at each point.
Danny Fell (33:22):
I think there’s a role for marketers to also be educators. I think we make a lot of assumptions about people’s knowledge of or comfort with analytics and data. And sometimes we just need to go back to some basics like: What do we mean by big data or thick data? What is an appropriate statistical model? Why do we use those models? So educating the marketing teams, as well as others in the organization, I think is part of that process as well, and I think that will tell you a lot about where people are on the spectrum of their comfort in using and getting value out of data, how marketing can work with other departments across the organization. What data, to your point, whether it’s clinical, or informational, or financial, what data are my other colleagues using that marketing should know about or would benefit from understanding? So I think that’s probably a big part of it as well.
Dean Browell (34:20):
I’d like just to add to that as an educator, is that then on the tail end of that, when you find some great insights, you go be an educator within the other departments. Other words, take them to them. Look, we found this interesting thing that included an employee subset, or whatever the case may be. Share the data and the insights that you ultimately pull together. That allows for a lot of great buy-in internally for you to come as the educator to these other departments. And it increases the level of collaboration and your access to their data by you … So sort of close that loop from an education standpoint. I think that could be really helpful too.
Alan Tam (34:55):
Yeah. Gentlemen, this has been an amazing conversation. I’ve learned a ton. It’s been super insightful. I’m sure many in the audience want to continue the conversation. What’s the best way for folks to continue the conversation with either of you? What’s the best way for folks to get ahold of you guys to chat about this some more?
Dean Browell (35:16):
I think LinkedIn for me.
Danny Fell (35:18):
Same.
Dean Browell (35:18):
Thankfully, there’s only Dean Browell, so it’s relatively easy. Should be easy to find.
Alan Tam (35:22):
Thank you so much. So for those of you in the audience that want to continue the conversation, these are two amazing thought leaders in data, qual and quant. I highly encourage you to reach out to either of them or both of them. Super insightful. There’s so much more to share, but unfortunately, we’re out of time for this podcast. Until next time, hello.
Outro (35:45):
If you want more of the latest from healthcare’s thought leaders, subscribe using the button below. Or you can visit hellohealthcare.com to get updates directly in your email.
Find the Clarity You’ve Been Missing
Learn how Actium Health is driving improved quality, outcomes, and revenue for innovative health systems nationwide.